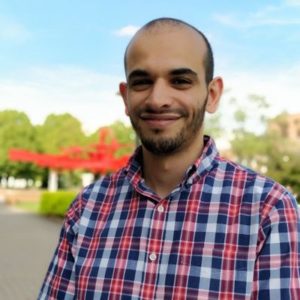
Hussein S. Al-Olimat
The following Great Innovative Idea is from Hussein S. Al-Olimat from The Ohio Center of Excellence in Knowledge-Enabled Computing (Kno.e.sis) at the Wright State University. Dr. Al-Olimat along with his coauthors Valerie L. Shalin, Krishnaprasad Thirunarayan, and Joy Prakash Sain were among the winners at the Computing Community Consortium (CCC) sponsored Blue Sky Ideas Track Competition at the ACM SIGSPATIAL International Conference on Advances in Geographic Information Systems 2019 (SIGSPATIAL 2019) in Chicago, IL. Their winning paper is called Towards Geocoding Spatial Expressions.
The Idea
The web and social media contain a vast amount of unstructured text with spatial referents. Meaningfully interpreting these referents by geocoding and localizing them is critical to support a wide-range of spatially-aware computing systems, including recommendation, search, and decision-support. Composite linguistic expressions encode location referents in natural language (e.g., “The area between X and Y is flooded.”). In our SIGSPATIAL 2019 vision paper, we introduce geocoding of such ad hoc spatial expressions that capture the richness of human location referents. The technique compensates for the current incomplete and imprecise geocoding methods that fail to unlock the potential of geocoding such location referents.
Coarse atomic toponyms (e.g., “Dayton, OH”) link to agreed-upon quantitative representations in locations gazetteers (e.g., OpenStreetMap). However, for interpreting inherently imprecise and unestablished areas (e.g., “north of Dayton”), such resources are inevitably incomplete. Specifically, humans use spatial expressions to capture unestablished regions using partial or finer toponyms (e.g., “Southern Ohio”) to refer to spatial areas qualitatively, making these expressions imprecise. Hence, we designed a tolerant formal representation that serves as an interlingua between these qualitative referents and their inherently fuzzy nature in terms of a precise quantitative system such as the World Geodetic System 1984 (WGS84). To geocode spatial referents, we identify and then operate on geographic extents (i.e., polygons) in a scalable manner in our formal representation. This perspective is formalized using rules and semantic approximations, and by exploiting background knowledge, including, for example, cultural knowledge concerning transportation (i.e., the availability of road network or the preferred mode of transportation) in expressions such as “6 hours south of Ohio”. Moreover, because we regard the semantics of locative expressions not to be entirely intrinsic, it cannot be adequately captured using a conventional approach through prepositions and the propositional content, so our representation incorporates pragmatics, by bringing in context (especially the effect of scale) that is extrinsic to a given sentence.
Impact
Geocoding ad hoc spatial expressions is essential for capturing the natural and accurate spatial context for practical context-aware computing system (e.g., used for disaster response). While the majority of systems rely on sensors data or generic spatial information drawn from atomic toponyms, they neglect the more accurate in-text ad hoc qualitative spatial expressions defining areas of location referents in question. Having this idea implemented will provide the needed infrastructure to support all kinds of context-aware computing and their visualizations that require more accurate location awareness and spatial contexts.
In the context of disaster response, if the mode of communication is a natural language, then location referents will most probably be imprecise, requiring further processing and mapping from the qualitative to imprecise quantitative representation, as mentioned above. For example, sending an ambulance to a specific area is essential for reducing overhead and cost, and for improving response time and efficiency.
Other Research
Our team, in collaboration with researchers from the civil engineering, geography, and computer science departments at the Ohio State University, have been developing state-of-the-art disaster management, forecasting, and recovery. We have integrated our collaborative work into prototypes providing storm surge modeling and flood extent prediction to generate forecasts about areas likely to be affected by disasters. Also, we employ multi-modal data and overlay them on real-time maps for enhanced situational awareness and to facilitate demand/request need matching during natural disasters.
Researcher’s Background
Since 2015, I have been a graduate researcher at the Ohio Center of Excellence in Knowledge-enabled Computing (Kno.e.sis) at Wright State University, Dayton, Ohio. At Kno.e.sis, I was the lead Ph.D. student on the NSF funded project “Hazards SEES: Social and Physical Sensing Enabled Decision Support for Disaster Management and Response”. Along with my other colleagues, I designed multifaceted syntactic, semantic, and pragmatic information extraction techniques, and my dissertation titled “Knowledge-enabled Information Extraction” has resulted in several publications in the areas of IE, NLP, and decision support. Our research has been implemented as open-source projects and has lead to ongoing collaborations with humanitarian and social development organizations.
Before joining Kno.e.sis, I finished my MSc in Computer Science from the University of Toledo, working on multi-objective optimization techniques using bio-inspired computing. In January 2020, I will be joining Tempus Inc., a biotech company, as an NLP scientist working on information extraction techniques for clinical discovery to support precision medicine research.
Links
For further information about my research, please visit my website. More information about our research group can be found here and here. Related research to the topic of our vision paper can be found here, here, and here.
To view more Great Innovative Ideas, please click here.